Feb '20 [tensor]werk Heartbeat

Hey Folks! This is our second [tensor]werk Heartbeat and we are sharing what happened during February on our side. Did you miss the first heartbeat?! Get yourself updated with the latest news and read it here.
Every month we are sharing news on projects we are working on, conferences and events we attend, what are our plans for the future and everything that might be related to data.
What’s going on?
We are working on a brand new design of our logo and website, in collaboration with Evoque. For the moment no spoilers, stay tuned on our channels 😉
We are introducing a few changes on the Hangar APIs: we are introducing the new columns
API, replacing the old arraysets
and metadata
terminology. With an arrayset
you could represent only tensors as a data type, while with a column
will be possible to represent also strings, replacing the functionality of metadata
in a way that is simpler, more effective and extensible. We foresee this being the last major API change before 1.0.
Hangar
We are dedicating this Heartbeat to our fastest-growing project: Hangar!
We briefly mentioned what Hangar is in the last Heartbeat. In this Heartbeat, we would like to provide a few more insights on what kind of problems it solves and some of the motivations behind it.
Hangar was born with the aim of simplifying data lifecycle operations in Machine Learning and Deep Learning workflows, allowing efficient versioning and collaboration on numerical data.
Organizations & projects commonly rely on storing data on disk in some domain-specific binary format (i.e. .jpg
images, .nii
neuroimaging informatics studies, .cvs
tabular data, etc.), and just deal with the hassle of maintaining all the infrastructure around reading, writing, transforming, and preprocessing these files into useable numerical data every time they want to interact with them. In fact, almost every data format requires a different program or a library to process them. This implies that most of the time people who work with data need knowledge about the data formats and the tools designed for them and not only about deep learning applied to some specific field (medical, agricultural, meteorological, etc.). On top of this, file-based representation of datasets is inherently rigid, when it comes to making such datasets evolve in time, collaborating over datasets or keeping multiple revisions of these datasets around for training or validation.
Hangar is designed to overcome these issues: we provide an API for storing and loading data in a standard and efficient way and also we aim at reducing the domain-specific knowledge gap required to work with different data types. When writing to a Hangar repository, you process the data into n-dimensional arrays once. Then when you retrieve it you are provided with the same array, in the same shape and datatype, already initialized in memory and ready to compute on instantly.
All this *wow* comes with an intelligent choice of the backend to be used to store your data: you won’t need to care about the best data storage system for your data. Based on heuristics, Hangar decides what is the best backend for the job, considering the arrayset specification (dimensionality, shape, sparseness, etc.) and system details. We are now supporting HDF5, Memmapped Arrays, TileDb and LMDB. As a user, this is completely transparent to you.
Hangar puts all these features behind a version control engine (based on a Merkle DAG, just like git). This enables data to be versioned, branched, merged and reconciled in case of conflicts. This means that we are able to time-travel along the history of data, know who did what on it, and access multiple branches in parallel, for instance for running multiple training jobs on different versions of a dataset at the same time.
Just like git, Hangar enables collaboration through remotes. A dataset can be cloned (that is, the repository information and commit history are copied from a remote, but not the actual data), fetched (the actual data is materialized), partially fetched (a subset of the data is materialized), pushed to and pulled from. In practice, this means that we can work on huge datasets stored on a remote, materialize a fraction of it (for instance to prototype a model on sample data) and push modifications to that fraction upstream.
Last but not least, Hangar seamlessly integrates with Machine Learning and Deep Learning workflows, through data loaders for PyTorch and TensorFlow. You can start training a model against data stored in a Hangar repo by literally adding one line to your existing code.
If you want to get started with Hangar, check out this brief tutorial from Jithin James 👉🏼 http://bit.ly/hangartutorial
Meet the people: Rick Izzo
Rick is Co-Founder and CTO of [tensor]werk, as well as the lead architect of Hangar. Prior to the founding of [tensor]werk in 2019, Rick was a Ph.D. candidate in Biomedical Engineering in the Endovascular Device Development Lab at SUNY Buffalo, Biomedical Engineer at the Jacobs Institute, fellow of the Prentice Family Foundation, and maintainer of the open-source Vascular Modeling Toolkit project (originally developed by fellow [tensor]werk Co-Founder, Luca Antiga).
He has expertise - and has published academic papers - in a wide a number of engineering, medical, and computer science domains including: Computational Fluid Dynamics, Medical Image Analysis & Segmentation, CAD for freeform anatomical model generation, Polymer-based multi-material 3D Printing, Interventional Cardiology (specific focus on transcatheter aortic/mitral valve replacement), Interventional Neurosurgery (catheter-based treatment of aneurysm, stroke, & pediatric hydrocephalus), storage, transformation, & computation on medical image datasets, and development & maintenance of open-source libraries written in Python & C++.
Outside of the lab, Rick is an amateur guitarist (primarily playing fingerstyle adaptations of bluegrass, classic rock, & anything by Mark Knopfler) and snow-skier. He is an avid traveler who loves to explore the world around him. He is currently well on his way towards a personal goal of traveling to 30 countries by the time he is 30 years old.
As CTO of [tensor]werk, Rick wrote an early prototype of the RedisAI runtime, and acts as lead architect and developer of Hangar. His current interests revolve around the efficient storage and usage of data (and how version control plays a role when curating and utilizing generalized datasets). His driving belief is that in the future (with tools like Hangar), the open-source software ethos will transfer into widespread open-source dataset curation and collaboration; allowing anyone who desires to generate insights from data collected about the world around us. We welcome anyone who connects with this belief to join our community and work!
Reach out
If you’d like to have a peek into our vision and our upcoming developments, please send us a note at info@tensorwerk.com. In any case, we will be posting our updates regularly here on Substack. Have fun and stay tuned.
If you want to stay up to date with ideas, projects and plans for the future at [tensor]werk, subscribe to our publication and receive the Heartbeat directly in your inbox.
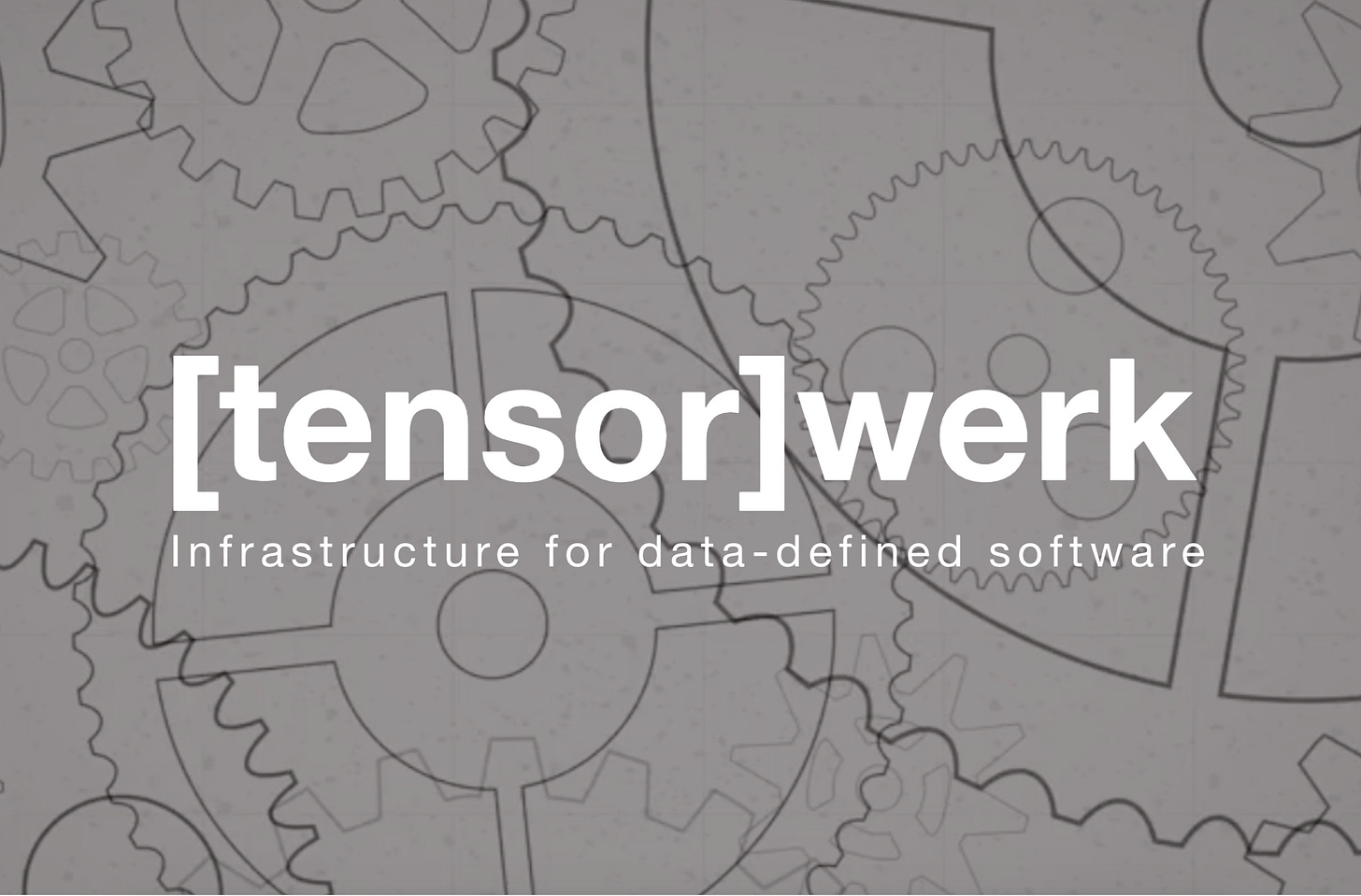