Mar '20 [tensor]werk Heartbeat

Hey there! While this SARS-CoV-2 global pandemic is affecting most of the world population, fortunately we were able to continue with our activities from NY, Italy and Bangalore and here comes the March’s Heartbeat to talk about one of our core products, RedisAI, and, hopefully, to take your mind off things.
Every month we are sharing news on projects we are working on, conferences and events we attend, what are our plans for the future and everything that might be related to data.
What’s going on?
We released Hangar 0.5! 🎉 As we mentioned in our Feb heartbeat, with this release we are introducing our last API breaking change, and for the better. In particular, you will find the new columns
API replacing the old arraysets
and metadata
terminology. With an arrayset
you could represent only tensors as a data type, while with a column
will be possible to represent also strings, replacing the functionality of metadata
in a way that is simpler, more effective and extensible.
This month we also submitted a PR to the MLflow project to support our RedisAI plugin, in order to seamlessly deploy MLflow models directly to RedisAI. Stay tuned for the updates!
RedisAI
While we briefly introduced RedisAI in our very first Heartbeat a couple of months ago, we would like to explain better what kind of problems it solves and why it’s a convenient tool to adopt in your stack.
Before diving into the details, let’s take a step back: what is Redis? Redis, which stands for Remote Dictionary Server, is a fast, open-source, in-memory key-value data store for use as a database, cache, message broker, and queue.
At a glance, RedisAI is a Redis module for serving tensors and executing deep learning models, born from a collaboration between [tensor]werk and RedisLabs. With the RedisAI module, Redis can store another data type, the Tensor.
As our CEO Luca Antiga use to say “Don't say AI until you productionize“, taking your deep learning model prototype out of that Jupyter Notebook and putting it to production is a critical but required step for real-world AI applications.
The strongest point on RedisAI is its easiness:
It is easy to work with models defined in different deep learning frameworks. In fact, RedisAI understands PyTorch and TensorFlow models directly, plus models saved in the ONNX interchange format (from almost any machine learning framework, including scikit-learn). RedisAI can actually execute models from multiple frameworks as part of a single pipeline.
It is easy to switch between devices you want your model to be executed on and there is no separate workflow for GPU or CPU.
It is easy to become an MLOps engineer: if you already have Redis in your stack, setting up RedisAI is a no-brainer and your DevOps engineers don’t need to learn anything else. Also because setting up RedisAI is a matter of 5-6 shell commands (or one Docker command 😉).
And since you are using Redis in the back, it is easy to scale your production runtime to a multi-node cluster setup with failover.
It is easy to manage the deployment even without Python - the language of choice of deep learning practitioners - if you don’t want to include it in your production tech stack! We have clients available for Python, Go and Java; for other languages, users can use native Redis Client libraries. Have a look at this repo for a showcase of Python, Go, Node.js and bash clients.
It is easy to reduce response time: RedisAI is architected in a way that it enables users to keep the data local, keep the model hot and keep the stack short. Fewer moving parts (data) mean less cost and fewer headaches.
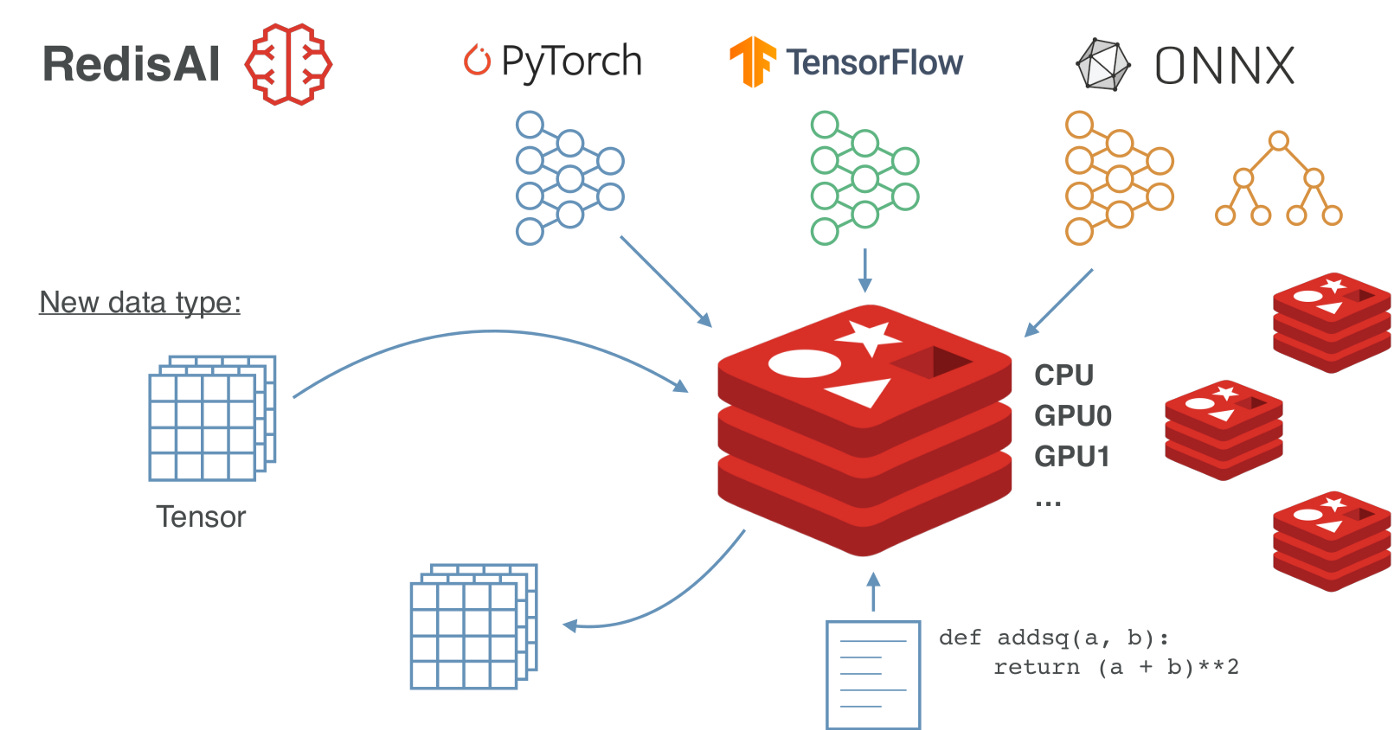
Oh, and not to mention that RedisAI is ~3 times faster than a REST API server a user would build using Django or Flask.
Although being written a year ago, read this blog post by our Sherin for a more comprehensive walkthrough of the features of RedisAI, including a comparison of existing deep learning runtimes, details on the installation and a practical example of an object detector with YOLO v3 step by step.
Meet the people: Sherin Thomas
Sherin, a.k.a hhsecond, is a senior developer at [tensor]werk started working with the team even before the company was founded. Sherin had spent his fair share of time on each tool [tensor]werk developed so far. He also created Stockroom, a high-level data + model + parameter versioning platform built on the foundation of Hangar and git. Sherin is expanding our super distributed team across the globe by working (and staying) from Bangalore. He is an author, speaker and also teaches students (and professionals) about programming in general and about different components in software 2.0.
Outside of work, Sherin is still a programmer 😁 He reads a lot (about a multitude of different topics spanning through psychology, tech, black holes, aliens, biology, management, etc), and is quite attached to the Bangalore startup ecosystem. He believes the current education system is still running with the decades-old methodologies and ideas and it should be rebuilt and hence helped to build fullstackengineering.ai. He is also fond of farming (although he never did farming, apparently) and probably use some AI stuff he copied from GitHub to build a robot to disrupt the Indian agriculture industry (that's what he says 😛).
Reach out
If you’d like to have a peek into our vision and our upcoming developments, please send us a note at info@tensorwerk.com. In any case, we will be posting our updates regularly here on Substack. Have fun and stay tuned.
If you want to stay up to date with ideas, projects and plans for the future at [tensor]werk, subscribe to our publication and receive the Heartbeat directly in your inbox.
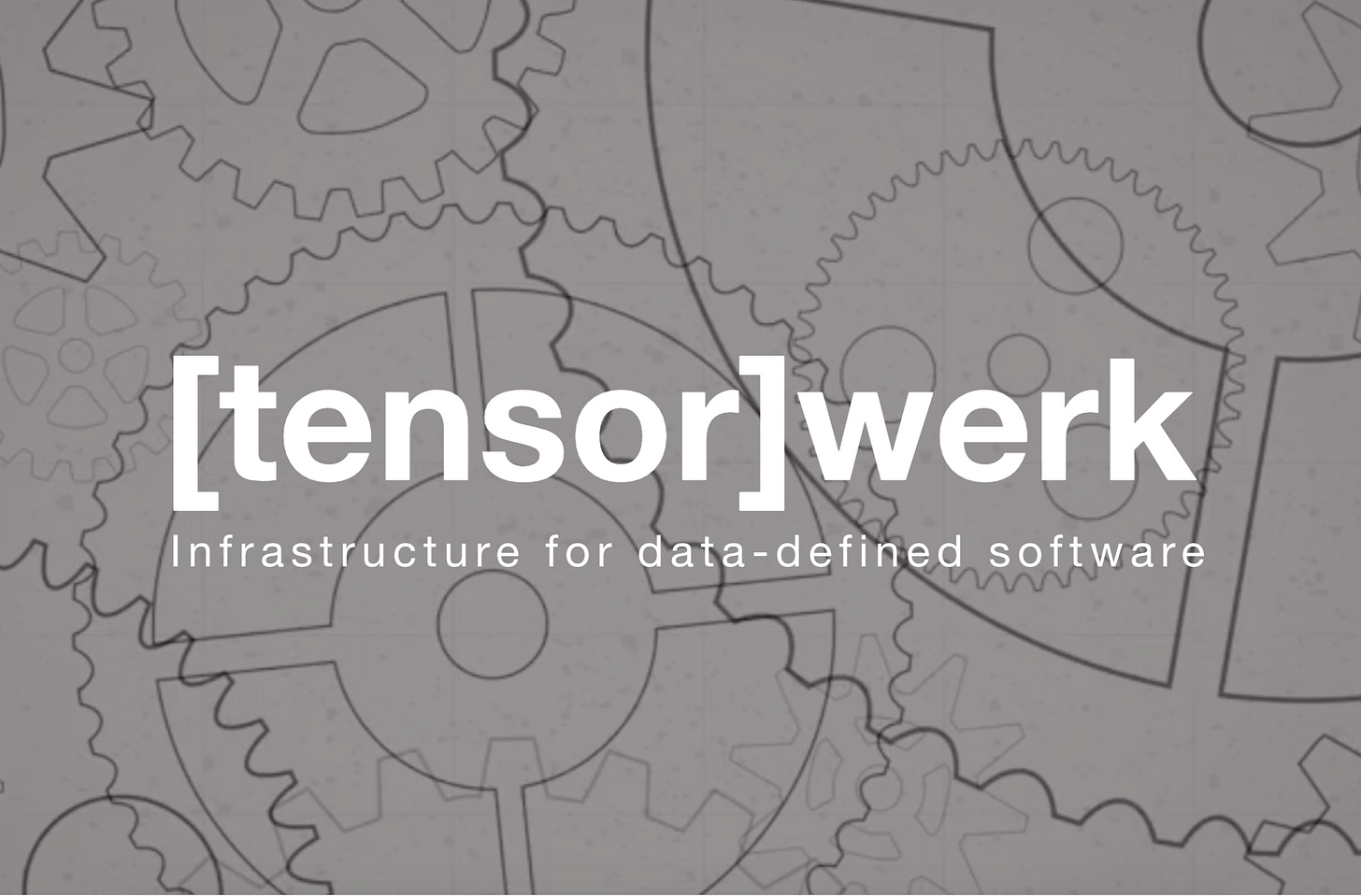